近日,華中農業(yè)大學食物經濟與管理團隊研究成果以“Dummy and effects coding variable in discrete choice analysis”為題在American Journal of Agricultural Economics發(fā)表。該論文以預包裝方便食品為實證案例研究了編碼方法的誤解對離散選擇研究分析的嚴重后果。
離散選擇實驗分析方法的廣泛應用已經迅速擴展到食物經濟、環(huán)境經濟、交通和城市規(guī)劃以及健康等諸多領域。選擇實驗方法可以用來估計以微觀經濟理論為基礎的偏好以及相關福利,然而估計的偏差和錯誤可能導致對經濟理論的誤解,引起錯誤的福利判斷,并進一步誤導私人和公共決策或政策的制定?;诖?,本研究對兩種屬性編碼方式,即“虛擬編碼”和“效果編碼”展開分析,概述了離散選擇分析中效果編碼方法的誤解。描述了效果編碼如何錯誤的處理和估計結果,進一步在消費偏好和福利分析中得出錯誤結論。
該研究分別從理論論證和案例研究的層面對效果編碼的誤解做出解釋。實證研究部分,論文設計了一個包含營養(yǎng)強化、可追溯、綠色食品標示等多個屬性的預包裝食品案例,并通過網上問卷方式獲取數(shù)據(jù)。理論推導和基于預包裝方便食品的實證研究結論均顯示,由于缺乏對兩種編碼方法之間等價性的理解,大量以前發(fā)表的文章都錯誤地解釋了效應編碼的結果。這種誤解會造成t檢驗結果、屬性偏好的大小和順序、邊際支付意愿以及消費者剩余/補償變化估計的無效。為解決編碼方法誤解的影響,本研究提出了一種轉換機制,可以在兩種編碼間轉換結果,并可以準確的計算和解釋參數(shù)估計和福利影響。最后,考慮到虛擬編碼產生的系數(shù)估計和相關福利測算比效果編碼解釋力度大、出錯率低,論文提出更推薦采用虛擬編碼方法處理選擇實驗的數(shù)據(jù)。該研究的結論不僅對離散選擇的計量經濟學分析做出貢獻,還糾正了包括食物經濟學領域在內的消費者福利分析和政策研究可能存在的潛在錯誤。
經濟管理學院青平教授為論文通訊作者,該研究的合作者包括原經濟管理學院博士后、湖北大學教育學院孫山老師以及來自美國俄亥俄州立大學和路易斯安那州立大學的學者。該研究得到了國家自然科學基金的資助。
【英文摘要】
Discrete choice models typically incorporate product/service attributes, many of which are categorical. Researchers code these attributes in one of two ways: dummy coding and effects coding. Whereas previous studies favor effects coding citing that it resolves con-founding between attributes, our analysis demonstrates that such confounding does not exist in either method, even when a choice model contains alternative specific constants. Furthermore, we show that because of the lack of understanding of the equivalence between the two coding methods, a sizeable number of previously published articles have misinterpreted effects coded results. The mis-interpretation generates conflicting preference ordering and renders t-statistics, marginal willingness to pay, as well as consumer surplus/compensating variation estimates invalid. We show that severe misinterpretation occurs for any categorical attribute that contains more than two discrete levels. The frequency of two-level attributes used in discrete choice analyses may have led some past studies to overlook this error. Given its equivalence and lower likelihood of misinterpretation, we recommend dummy coding.
論文鏈接:https://doi.org/10.1111/ajae.12311
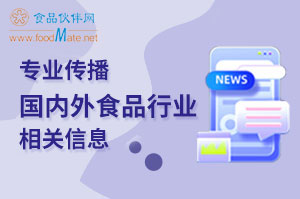