近日,華中農(nóng)業(yè)大學柑橘全程機械化平臺研究團隊以“Non-destructive fruit firmness evaluation using a soft gripper and vision-based tactile sensing”為題在農(nóng)業(yè)計算機與電子信息領域期刊Computers and Electronics in Agriculture發(fā)表研究論文。該研究通過視觸覺傳感柔性末端機械爪與深度學習方法,實現(xiàn)了對球形水果的穩(wěn)定抓取和成熟度準確分類。
果實硬度是判斷其成熟程度的重要指標之一,反映了內部淀粉物質的形態(tài)變化,可以更準確地指示內部組織的成熟度,對其準確評估對采后管理決策具有重要參考意義。團隊受到鰭條效應啟發(fā)設計了一種軟材料制作的柔性機械爪,其具有穩(wěn)定無損抓取水果的功能的同時,可聯(lián)合深度學習網(wǎng)絡評估抓取過程中的作用力大小,進而實現(xiàn)判斷水果硬度和成熟程度的功能。
研究首先使用有限元建模和相關實驗證明了柔性材料制作的機械爪具備實現(xiàn)穩(wěn)定抓取同時、兼具非破壞性的優(yōu)勢;隨后結合視覺技術記錄機械爪與水果接觸時的變形特性,提出的深度學習網(wǎng)絡的序列式圖像信息處理模型——包括卷積神經(jīng)網(wǎng)絡、長短時記憶單元,使得該網(wǎng)絡模型能夠將軟機械爪在時序下的變形特征,進行作用力分析進而推斷水果硬度與成熟度。研究以西紅柿和油桃為試驗樣本,驗證了柔性機械爪的抓取穩(wěn)定性,并采集了相關序列圖像數(shù)據(jù)集與水果硬度預測的深度學習網(wǎng)絡。
同時為了方便現(xiàn)實場景中的應用,研究團隊將控制算法和深度學習模型移植到了邊緣計算設備上,并將該套系統(tǒng)搭載在機械臂上,提高了運動自由度,預期可實現(xiàn)生產(chǎn)線上的基于硬度的水果成熟度分級。同時制作成本低廉,準確度較高,與其他水果硬度與成熟度檢測手段相比應用潛力巨大。
我校工學院博士研究生林家豪和本科生胡晴為本論文共同第一作者,工學院副研究員陳耀暉和澳洲聯(lián)邦科學與工業(yè)研究組織博士后研究員王興為共同通訊作者。工學院李善軍教授參與了論文的指導工作,碩士杜璇和理學院碩士趙亮共同參與了論文研究。
【英文摘要】
As fruit firmness is a crucial characteristic associated with the maturity level, its accurate estimation is of great importance to post-harvest processing and wholesale in the industry. Benefiting from the advances of soft robotics, a soft gripper with simultaneous compliant deformation and tactile sensing is proposed in this study for the fruit firmness classification. The gripper design inspired by the fin ray effect can achieve active deformation, which helps simplify the actuation system and improve the delicate manipulation capability. Finite element modelling, along with experimental tests, is first utilized to validate the gripper's feasibility in compliant and safe fruit grasping, and respiratory tests are then conducted to further demonstrate the non-destructive nature. Moreover, fruit–gripper interaction is captured by visual sensors and then processed using an attention-based CNN–LSTM algorithm to predict firmness information. Tomatoes and nectarines are chosen as the sample fruit for experimental validation. R? values of their firmness prediction are 0.795 and 0.753, and the accuracy of maturity grading is 84.6% and 81.5%, respectively. In general, the soft gripper provides a promising solution for both safe grasping and non-destructive firmness evaluation, and it is expected to be integrated into automated production lines to pack fruit based on different firmness levels.
論文鏈接:https://www.sciencedirect.com/science/article/pii/S0168169923006440
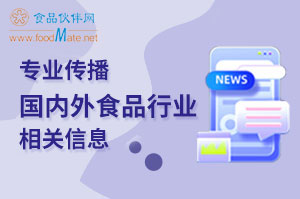