近日,華中農業(yè)大學工學院王巧華教授團隊研究成果以“Rapid identification of A1 and A2 milk based on the combination of mid-infrared spectroscopy and chemometrics”為題在Food Control發(fā)表。研究揭示了A1和A2牛奶的光譜差異,建立了無損檢測兩種奶的分類模型,表明中紅外光譜技術可作為無損快速鑒別A1奶和A2奶的新工具。該研究也可為單獨組建A1型和A2型奶牛育種群提供相應的技術支持。
僅含A2β-酪蛋白的牛奶(A2奶)因其獨特的健康益處而在全球廣受歡迎。長期以來,企業(yè)需要先對奶牛進行專業(yè)的基因檢測,篩選出β-酪蛋白中只包含A2β-酪蛋白的純種A2奶牛,再用這些奶牛生產的牛乳加工成A2奶?;驒z測雖準確性高,但成本也高且耗時長,無法滿足乳企規(guī)模化生產的要求。因此,急需開發(fā)一種低成本、高效益的技術快速識別A1奶(普通奶)和A2奶。該研究突破了傳統基因檢測的局限,應用中紅外光譜技術快速鑒別出A1和A2牛奶。

CARS算法篩選特征變量
該研究分析A1和A2牛奶在中紅外光譜吸光度上的差異,找到敏感波段組合作為全光譜,分別利用標準正態(tài)變量變換 、多元散射校正、歸一化、一階導數、二階導數、一階差分和二階差分等7種方法對光譜進行預處理,利用無信息變量消除法和競爭性自適應重加權算法篩選出能代表A1和A2奶差異的特征變量,進而構建偏最小二乘判別分析(PLS-DA)模型和支持向量機(SVM)模型,PLS-DA模型的訓練集準確率和測試集準確率分別為96.6%和96.0%,SVM模型的訓練集準確率和測試集準確率分別為96.0%和95.1%。

UVE算法篩選特征變量
該研究選擇PLS-DA模型作為最佳模型,使用一組獨立樣本對模型進行外部驗證。將新采集的牛奶中紅外光譜批量帶入保存的模型中,以對應的奶?;驒z測結果作為對照指標,模型的預測準確率為95.2%,性能良好。結果表明,中紅外光譜技術可以實現對A1奶與A2奶的快速分類鑒別,有望將來在生產中得到應用。
華中農業(yè)大學工學院碩士研究生肖仕杰為論文第一作者,工學院王巧華教授和動物科學技術學院張淑君教授為共同通訊作者。該研究得到中國政府項目(2013070204020045)資助。
【英文摘要】
The milk containing only A2 β-casein (called A2 milk) is globally popular because of its unique health benefits. Traditionally, genetic testing (such as gene sequencing) is used to identify the cows with A2 β-casein gene that can only produce A2 milk, which is a time-consuming and costly method. The objective of this study was to directly identify A1 and A2 milk from a large quantity of milk using mid-infrared (MIR) spectroscopy and chemometrics without genotyping cows. Before establishing the predictive model, we firstly genotyped the A1 β-casein and A2 β-casein of cows from blood as reference values. Further, the MIR spectra of the milk collected from these cows were obtained using a dairy product analyzer. The MIR spectroscopy data and the reference values were used as the independent and dependent variables, respectively, to establish a category classification model for A1 and A2 milk. Seven preprocessing methods were combined with two feature extraction algorithms to establish the model. Subsequently, partial least squares discriminant analysis (PLS-DA) and support vector machine (SVM) models were developed. The average accuracy of the test set of the two models were 94.9% and 94.4%, respectively, while the PLS-DA model exhibited better effect, and the accuracy of training set and test set reached 96.6% and 96.0%, respectively. We used a set of independent samples for the external validation of the PLS-DA model, and the prediction accuracy was 95.2%. Overall, the proposed prediction models based on MIR spectroscopy can be used for low-cost, rapid, and large-scale classification of A1 and A2 milk, which may be extremely beneficial in milk production industries.
論文鏈接:https://doi.org/10.1016/j.foodcont.2021.108659
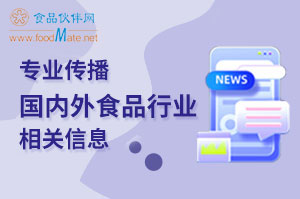