近日,華中農(nóng)業(yè)大學(xué)柑橘全程機(jī)械化平臺(tái)和人工智能與統(tǒng)計(jì)學(xué)習(xí)團(tuán)隊(duì)以“Predicting and Visualizing Citrus Colour Transformation Using a Deep Mask-Guided Generative Network”為題在農(nóng)藝學(xué)領(lǐng)域期刊Plant Phenomics發(fā)表研究論文。該研究通過生成式深度學(xué)習(xí)方法,實(shí)現(xiàn)了對(duì)柑橘果皮顏色變化的高精度可視化預(yù)測(cè)。
柑橘果皮顏色是果實(shí)發(fā)育的良好指標(biāo),因此監(jiān)測(cè)和預(yù)測(cè)其顏色變化可以幫助農(nóng)作物的管理和收獲進(jìn)行決策。研究觀察了107個(gè)臍橙樣本,在其果皮顏色轉(zhuǎn)變期間采集了7535張柑橘圖像,構(gòu)建了首個(gè)柑橘轉(zhuǎn)色數(shù)據(jù)集。研究提出了一種將視覺感知融入深度學(xué)習(xí)的網(wǎng)絡(luò)框架,包括分割網(wǎng)絡(luò)、生成網(wǎng)絡(luò)和感知損失網(wǎng)絡(luò);生成網(wǎng)絡(luò)中的嵌入層將圖像特征和時(shí)間信息進(jìn)行了有效融合,使得該網(wǎng)絡(luò)模型能夠根據(jù)輸入圖像和不同的時(shí)間間隔來生成果皮顏色轉(zhuǎn)變的預(yù)測(cè)圖像。
為了方便現(xiàn)實(shí)場(chǎng)景中的應(yīng)用,研究團(tuán)隊(duì)將該模型移植到了安卓設(shè)備APP上,通過手機(jī)相機(jī)拍攝柑橘圖像,并在APP中輸入感興趣的時(shí)間間隔即可完成預(yù)測(cè)。該研究成果預(yù)測(cè)柑橘果皮顏色的變化并使其可視化,為柑橘果園管理實(shí)踐提供有效幫助,并為其他水果作物的研究提供了借鑒和擴(kuò)展的可能性。
我校理學(xué)院碩士研究生鮑澤韓和副教授李偉夫?yàn)楸菊撐墓餐谝蛔髡?,工學(xué)院副研究員陳耀暉和海南大學(xué)生物醫(yī)學(xué)工程學(xué)院副教授肖馳為共同通訊作者。理學(xué)院教授陳洪和日本RIKEN研究所研究員Vijay John參與了論文的研究和指導(dǎo)工作。
【英文摘要】
Citrus rind colour is a good indicator of fruit development, and methods to monitor and predict colour transformation therefore help the decisions of crop management practices and harvest schedules. This work presents the complete workflow to predict and visualize citrus colour transformation in the orchard featuring high accuracy and fidelity. 107 sample Navel oranges were observed during the colour transformation period, resulting in a dataset containing 7535 citrus images. A framework is proposed that integrates visual saliency into deep learning, and it consists of a segmentation network, a deep mask-guided generative network, and a loss network with manually-designed loss functions. Moreover, the fusion of image features and temporal information enables one single model to predict the rind colour at different time intervals, thus effectively shrinking the number of model parameters. The semantic segmentation network of the framework achieves the mean intersection over union score of 0.9694, and the generative network obtains a peak signal-to-noise ratio of 30.01 and a mean local style loss score of 2.710, which indicate both high quality and similarity of the generated images and are also consistent with human perception. To ease the applications in the real world, the model is ported to an Android-based application for mobile devices. The methods can be readily expanded to other fruit crops with a colour transformation period. The dataset and the source code are publicly available at Github.
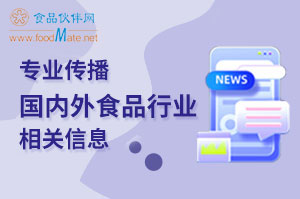